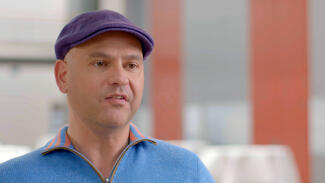
Chris Armbruster: Die Data Scientists von morgen
Die deutsche Industrie sucht sie händeringend: Data Scientists, also Experten im Umgang mit großen Datenmengen. Aber woher nehmen? Im Moment gibt es noch goldene Zeiten für Bewerber.
"Students have done things that we had never anticipated with the knowledge that they have taken from our data science courses."
Video abspielenVon wegen lästige Pflichtveranstaltung: In Berkeley, an der Top-Universität Kaliforniens, hat man schon vor Jahren einen Grundkurs für alle Studierenden eingeführt, in dem sie lernen, Daten richtig auszuwerten. Und der Kurs ist ein Riesenerfolg, so die Programmleiterin Cathryn Carson. Warum ist das so – und was hat das auch sonst der Uni gebracht hat?
The University of Berkeley introduced a data science course for early-level students a few years ago. And it has become a great success. Cathryn Carson, Operational Lead of the Data Science Education Program at UC Berkeley, tells why – and how the university as a whole has benefitted from it alongside.
Zum Thema Datenkompetenz hat der Stifterverband eine Förderinitiative speziell für Hochschulen gestartet: Data Literacy Education.
Jede Woche neu beim Stifterverband:
Die Zukunftsmacher und ihre Visionen für Bildung und Ausbildung, Forschung und Technik
Autorin: Corina Niebuhr
Produktion: Webclip Medien Berlin
für den YouTube-Kanal des Stifterverbandes
The students bring the enthusiasm and the motivation. The university brings the training, and then the partners bring the data sets. Bringing this all together in a common setting makes it possible through the students to bring about the facts in the world that are so motivating.
Students who go out into the world with data science skills, with data literacy at their disposal are able to bring those skills to bear on the societal challenges ahead of us. And when they are learning to think both technically and ethically to understand how to work the data and what it should be used for, they give us the possiblity of shaping a more just society around data.
Our students today are living in a world that's just saturated with data. And that can lead them sometimes to just see themselves as consumers of their products. But when their eyes are open to the fact that they can become producers of knowledge in the areas that they find interesting, their attitude towards data science can shift.
Students have gone on and done things that we had never anticipated with the knowledge that they have taken from our data science courses. Some of them have gone on into what we call discovery projects which are team projects put together either by faculty members or by outside partners, non-governmental organisations, grand data challenges that students come together, join their skills and work on around things like the availability of safe drinking water or pollution control. And there's no way that the faculty could have told the students, this was what they should do with their skills. It engaged the students and they jumped at it. Or else they have gone and worked in faculty and many different parts of the university to do research projects inside laboratories. And the faculty and the postdoctoral fellows tell us: The students bring skills that we don't ourselves have. We can do new science when we bring in your students and they become part of our lab team as equal contributors.
So data literacy is an emerging demand on our society. We haven't had the same need for it before like we're seeing now. Because the wealth of data that is surrounding us and the way we use it to make personal decisions is placing new demands on our educational system. So the possibility of spuring innovation in this area, finding ways to make it available to students across the spectrum of disciplines is an incredibly important move.
The feedback on the programme has been really encouraging for students. Many of them come in to Data 8 and the Connector just to meet a requirement, a statistics requirement. And then they take the class and they say: I never realized data science could be for me. I can be data science. So as the students take it up they internalize it and make it their own. And as a professor that's one of the most exciting things you can see is the thing that you know was going to be so valuable they feel is theirs. And on the side of my colleagues at the faculty who are themselves learning how to deal with data science, what we have been able to do is bring that student energy to work with them. And the students bring the knowledge from Data 8 to faculty who haven't had that experience themselves. So in the module, say, a student from computer science will be working with the faculty member from sociology, psychology and bringing these new ways of working with data directly to the faculty member and into that faculty member's courses. And it's a cross-generational partnership where the knowledge is being shared in both directions.
So at Berkeley we started down this road back in 2014. So it's been four years coming. A group of us faculty came together in order to design a very, very ambitious undergraduate curriculum, designing it surely for the pleasure of working in this area and knowing that our students would want it. So as this group has come together and continued working and expanding, bringing in other faculty from across the university, we have not only built the foundations of data science class what we call Data 8 which is now running at 1,300 students every semester, but a whole set of other courses around it. At the entry level we have got Connector courses which bring the concepts and skills of Data 8 into connection with all sorts of domains of application, from text analysis to civil engineering. And then as we have moved upward in the curriculum we found that we can build new courses or transform existing ones by bringing data science modules in, so sociology classes use data science, or environmental science classes. It's really exciting to be part of something that is working from the bottom up and building collaborative relationships across the university rather than silos.
There was a kind of insurgent ideal that motivated those of us who were involved with the programme from the start. We knew that data science needed to get into domains all across the university, and we knew it needed to be on those domains' terms. You can't dictate from above how this will unfold. So we started at the bottom with the foundational level and with the students and then made it possible for all the application domains to see themselves in the programme they were building and define their role that made the most sense for them, whether that's computer science and statistics being all in and being there at the foundations or whether its faculty in the natural sciences or the humanities creating new Connector courses or new modules. We make space for every discipline to find its own way, and that was part of the goal from the start.
This needn't be a daunting task. There are small ways to start, experimental ways to start, whether it is teaching a new class or teaching a class in a new way. It doesn't need to be grandiose at the beginning. The opportunities here also come from working with colleagues in new more exploratory ways, not creating a grant proposal that will have to go through many layers of review but just sitting down with a good colleague who you trust and developing a relationship and strategy for working together. One of the things that have been so surprising for me, that's most valuable about the pay-off is the personal relationships that come out of working with colleagues. The friends who I've made through this effort are going to stay with me for years. And that's because you get past your barriers, and you get past your disciplinary pride, and you find ways to engage with someone else's expertise. So it can start small and it can be experimental. But with a strong personal relationship it can grow into something really powerful.